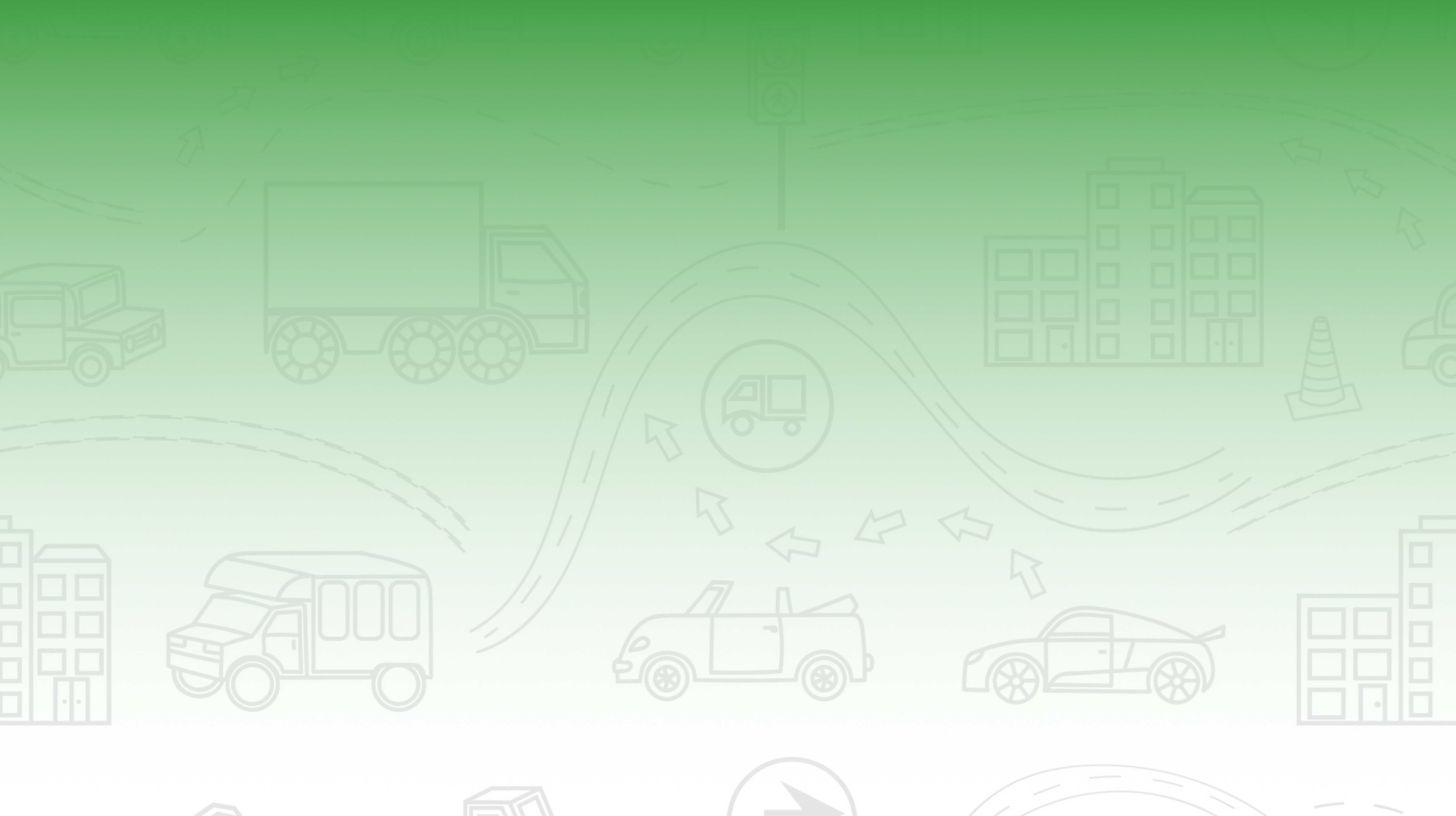

Our work behind an automotive company successful exit: 52k dealerships and 10M cars a day.
A real-time used-car community that connects dealers and consumers with a live team of expert appraisers to create transparency and efficiency in the trade-in process.
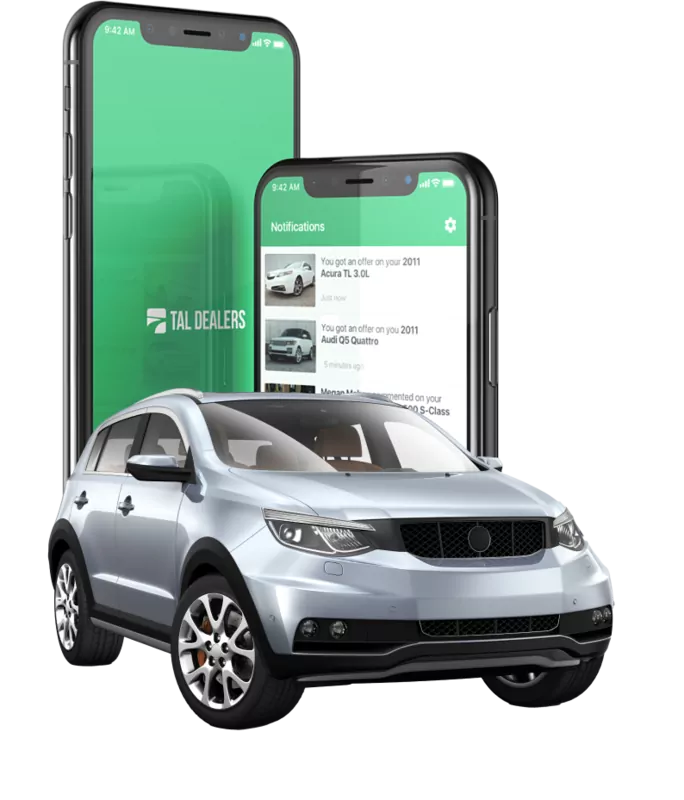
Challenge
Provide real-time market insight for the American automotive industry. Visit more than 52.000 US car dealer websites and obtain more than 10 million car data points each day in a performant way.
The client wanted to build a Ruby on Rails platform that provided them with real-time analytics.
This included information about:
- Car movements between dealerships (either transfers or direct sales)
- Which makes and models were being sold the most
- At which price and time they were being sold
- Heatmaps of where most of those sales were being done
- Detect which cars are harder to sell, among others.
Any customer could later run business analytics on a certain US region, for a certain kind of car, to figure out specific properties about that market. Using this information they could improve their marketing effort, detect tendencies, and most importantly improve the accuracy of their appraise (so it’s closer to the real market price).
The main challenge was building a massive parallel data acquisition platform in Python that could handle obtaining data points from 10 million vehicles each day in a cost-effective way. Performance was a priority from the ground up since we wanted to obtain as much data as possible, with as few AWS EC2 instances as possible.
Also, we needed to store, process, and make accessible (ETL) all that daily data for later usage (billions of car data points). This data needed to be queried in real time, so this was another important challenge to tackle.
All the work that we did on Wolfy gave us the experience and know-how to tackle this big technical challenge that we had. Just as we thought, having founded our own tech product would give us more tools to help other startups with their challenges.
Solution
We built a massive parallel data acquisition platform in Python and a data pipeline to process and store the information.
We built a distributed master-slave architecture using Python as the main programming language. Scrapy and Selenium to automate the browser processes, and Frontera for implementing a frontier.
Every process was containerized and managed using Kubernetes, all running on AWS's EKS. The solution was deployed using multiple AWS services like EKS, ECR, EC2, Elastic Search Service, Redis Service, RDS, S3 among others.
A master-slave architecture was implemented so that every day the master process would spin up several EC2 machines with several slave processes each. When each process was finished, the master process would stop the idle instances to avoid extra costs.
All the obtained data was saved to a RDS database that we later dumped to S3 files (as a backup).
Finally, we used the S3 dumps to perform an ETL process to load the obtained data into an Elasticsearch search engine. Using the processed data from Elasticsearch, we exposed all the important information through an API that customers could easily query.
The client had billions of vehicle data points generated by this process, and they could query them to gain real-time insight into the market data.
Outcome
A scalable and cost-effective platform, where the data pipeline and ETL process could handle and process billions of data points. The ETL process we created integrated with some of the client's existing data and we were able to process and store more than 10 million data points per day (that’s more than 3.5 billion per year!).
Users were able to create custom API calls that queried billions of car data points (with sub-second response times) to get real-time information about the market and improve their sales process.
As a consequence of the value they were giving to their users, our client had a successful exit and was bought by a very important player in the field.
This was one of our first clients where we had to integrate with their existing team and processes. It was a pleasure to work with our first automotive startup!
Check out our case study of Tradex, one of our current partners from the automotive industry.
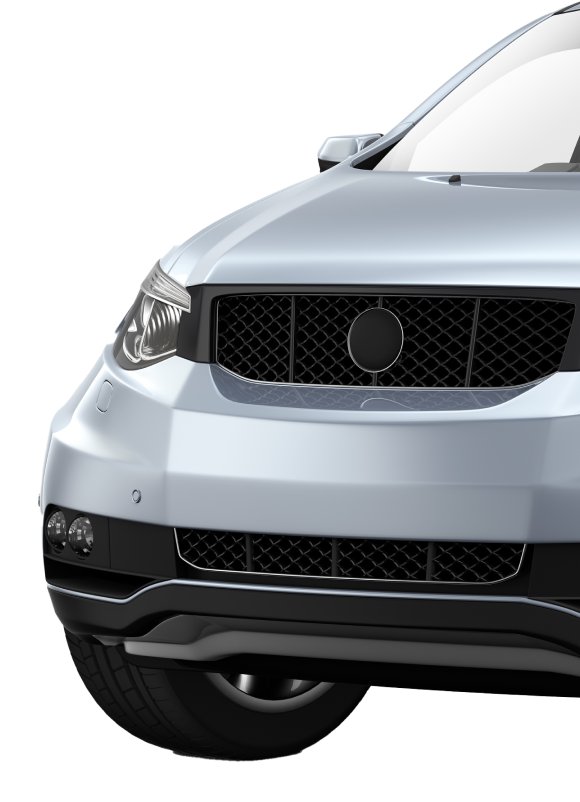
Toolbox
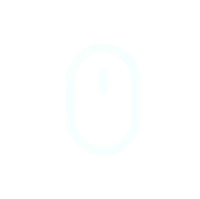
Tech
Ruby on Rails & Python
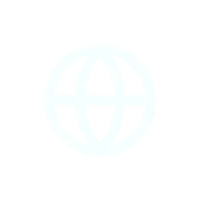
Solution
Massive scraper and web platform
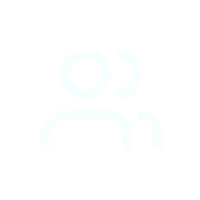
Team
6 Developers